IQMR 2024 will run from June 16-28
participants
nominating institutions
weeks
How to participate
Open Pool
There are four separate open pool competitions to attend IQMR 2024: the general open pool; applications from researchers at African scholarly institutions; applications from researchers at Latin American scholarly institutions; and applications from scholars based in the Arab MENA region. You can find links to the separate application forms in the table below.
Scholars admitted through the general track will have their participation at IQMR covered, receive a stipend to contribute towards cost of meals, as well as shared-accommodation for the duration of the program. General track attendees will be responsible for their own transportation costs to and from Syracuse University. Scholars admitted through the African, Latin American and MENA tracks will have participation at IQMR covered, meals, and shared-accommodation for the duration of the program, as well as roundtrip economy-class airfare.
Nomination
Distinct from the open pool process, IQMR also accepts attendees who are nominated to attend by their home unit (center/department/school) using their own selection procedures. The participation fee varies depending on the number of people being nominated. For 2024, the participation fee per nominee is $1670 if one, $1640 if two, $1620 if three, $1600 if four, and $1580 if five. The participation may be paid by either the nominating home unit, or by the nominee. If you have any questions about the nominating process, please contact Colin Elman at celman@syr.edu.
IQMR 2024 will offer nominees a range of lodging options with different costs. More details will be posted here when those arrangements have been finalized.
Application Information
General
Application deadline: 10/23/23
Number of slots: 15
Travel bursary: No
Part, room and board: Yes
Arab MENA
Number of slots: 4
Travel bursary: Yes
Part, room and board: Yes
Latin America
Application deadline: 10/23/23
Number of slots: 5
Travel bursary: Yes
Part, room and board: Yes
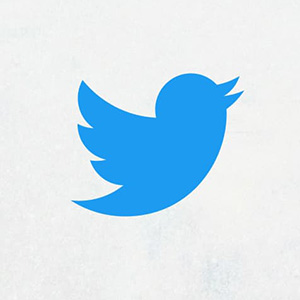
The Ethnography module at #IQMR2021 has me 🤯 over and over again. I feel like even the questions I didn't know I wanted to ask have been answered, and the description/reflexivity/analysis chart is going straight in my toolbelt. Thank You!" via Twitter.
Piper Biery @Piper_Biery
Hear From Our Participants
Learn to create and critique methodologically sophisticated qualitative research designs, including case studies, tests of necessity or sufficiency, and narrative or interpretive work. Explore the techniques, uses, strengths, and limitations of these methods, while emphasizing their relationships with alternative approaches. And receive constructive feedback on your own qualitative research designs.
Watch our YouTube playlist for video introductions to each of the conference sessions.
New Voices Initiative
IQMR’s New Voices Initiative aims to identify, encourage and support early career researchers (ECRs) who are interested in teaching at IQMR. Our hope is that each fall several ECRs will be selected through a transparent, competitive process, and will join the teaching team for particular module sequences during the following summer’s institute. Seasoned instructors will guide and mentor ECRs who are selected, and will learn from and draw on their fresh insights and perspectives about the methods being taught. The initiative’s broader goals are to grow the number and diversity of faculty who teach qualitative and multi-method research in the social sciences. As part of the New Voices Initiative, three ECRs were selected as teaching fellows at IQMR 2023.
IQMR 2023 ECR Teaching Fellows:
Please note that while we are hopeful that participants in IQMR 2024 will be able to gather in Syracuse next summer, we realize that this will ultimately be dictated by health circumstances. If we are unable to hold an in-person institute, IQMR 2024 will take place online. We will offer details regarding specific arrangements early next year once we have further information on the progression of the COVID pandemic, and faculty and student needs.